Impact Assessments on Agricultural Productivity of Land-Use Change
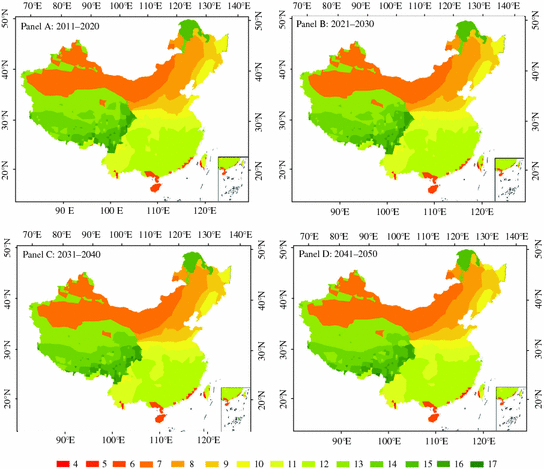
Fig. 2.1
Land-use pattern of the North China Plain in the year of 2008
However, the rapid population growth and urban land expansion have led to serious cultivated land loss and environmental degradation in the North China Plain. Many researches showed that the conversion from cultivated land to other types of land led to the decrease of cultivated land in the North China Plain (Jiang et al. 2011) pointed out that the area of cropland abandonment was much greater than the area of cropland reclamation in the North China Plain (Jiang et al. 2011; Shi et al. 2013). Besides, the quality of cultivated land converted to other land-use types is generally higher than that of the cultivated land converted from other land-use types. As a result, the shrinkage of cultivated land in the North China Plain will exert great impact on the land quality. How to improve land productivity is becoming vital for the improvement of agricultural production. In addition, though the statistic data showed that the grain yields were increasing, it is due to the additional investment into the agricultural production. With this regard, it is an urgent issue to study how to make scientific policy and sustainable land management measures to rationally regulate the land conversion and control land degradation to improve the productivity of the remaining resources in the agricultural sector.
Data Preparation
According to previous studies, driving factors of land degradation are complex. Among which, biophysical causes (include topography that determines soil erosion hazard, and climatic conditions, such as rainfall and temperature) and land-use change (such as deforestation, desertification resulted from unsustainable land management practices) are major direct contributors to land degradation, while the underlying causes of land degradation include population density, economic development, land tenure, and access to agricultural extension, infrastructure, etc. Thus, in order to analyze the situation of agricultural production and impact mechanisms of climate and land-use change integrated with socioeconomic factors on cultivated land degradation in the North China Plain, we collected the NDVI data, land-use datasets, climatic data, socioeconomic statistical data, and other geophysical data of the year of 2000–2008.
NDVI. NDVI is one of the most commonly used indicators for vegetation monitoring (Tucker 1979). Many previous studies have used the NDVI and other measures based on the NDVI as indicators of changes in ecosystem productivity and land degradation, which showed that NDVI is strongly correlated with the green vegetation coverage (Elhag and Walker 2009). Though there are some limitations and criticisms related to the use of this measure, NDVI remains the only dataset available at the global level and the only dataset that reliably provides information about the condition of the aboveground biomass (Nkonya et al. 2011). In this study, we used the remote sensing data of NDVI to measure the land degradation. Before 2006, the NDVI data were obtained from the GIMMS NDVI dataset produced by the GLCF (Global Land Cover Facility) research group at the University of Maryland from July 1981 to December 2006, with a spatial resolution of 8 km by 8 km and a temporal resolution of 15 days. The NDVI data after the year 2006 were derived from SPOT VEGETATION data (ten-day ensembles) which were derived from a dataset developed over the period from April 1998 to December 2010, with a spatial resolution of l km by l km. Based on the period when the two datasets overlapped (1998–2006), we conducted data processing according to the previous experience of Yin et al. (2014). Firstly, correlation analysis between the maximum monthly NDVI and other factors was performed, following which a linear regression equation was established to extend the Global Inventory Modeling and Mapping Studies (GIMMS) dataset from 2007 to 2008. This helps to eliminate any sensor error in the other two datasets. To ensure the data quality, reliable and internationally recognized data pretreatment processes were used. In order to eliminate cloud contamination effects and the noise caused by other atmospheric phenomena, we included a smoothing method proposed by Chen et al. (2004) based on the Savitzky-Golay filter. Finally, we clipped the NDVI data of the North China Plain during the period from the year of 2000 to 2008.
Land use. The land-use data came from the land-use database of the Resources and Environment Scientific Data Center, Chinese Academy of Sciences (Liu et al. 2010). The database was constructed from remotely sensed digital images by the US Landsat TM/ETM satellite with a spatial resolution of 30 by 30 m. Further the land-use data are upscaled to 1 by 1 km. The data that were analyzed in this study covered the year of 2000 and 2008.
Climatic data. The climatic data, mainly including temperature and rainfall from the year of 2000 to 2008, were derived from the daily records of 117 observation stations covering the entire area of the North China Plain, which were maintained by the China Meteorological Administration. A spline interpolation using the coupling-fitted thin-plate interpolation method was chosen to interpolate the meteorological data. Based on the climatic dependence on topography, climatic variables were interpolated with spatial resolution of 1 by l km. At a broader scale, temperature declined roughly with the increasing elevation at a typical standard lapse rate of 6.5 °C per 1 km, so the interpolated air temperature data were adjusted to the sea level according to the altitudinal correction factors based on the digital elevation model (DEM) dataset.
Soil organic matter. The soil fertility map of the study area was clipped from the soil map of China provided by the Institute of Soil Science, Chinese Academy of Sciences. It was based on the maps on the scale of 1:4,000,000 from the Second National Soil Survey of China for Soil Organic Matter (SOM).
Socioeconomic statistical data. In this study, we collected and processed the socioeconomic data of 385 major counties in the North China Plain at a county level for the period of the year of 2000–2008, including the gross domestic production (GDP), population, rural farmers’ per capita income, fertilizer utilization, pesticide utilization, farm machinery, total grain production, and total grain sown area, which were all acquired from socioeconomic statistical yearbooks published by National Statistical Bureau of China.
Geophysical data. The geophysical data are also closely related with the land productivity. In this specific study, the geographic factors mainly include elevation and average slope, the data of which were derived from China’s Digital Elevation Model Dataset supplied by the National Geomatics Center of China. Another part of the geophysical data was the proximity variables, including the distance from each grid to the nearest expressway or highway, and other way, which were incorporated to measure the impacts of the infrastructure construction on the land degradation.
Primary Analysis
Changes of grain production. We analyzed the changes of grain production and the relationship between total grain production and grain yield (kg/ha) of the North China Plain during the year of 2000–2008. The spatial distribution of grain yield at municipality or county level in the year of 2000, 2005, and 2008 is shown in Fig. 2.2. The grains mainly included wheat and maize, but some rice, soybean, and tuber crops were also included.
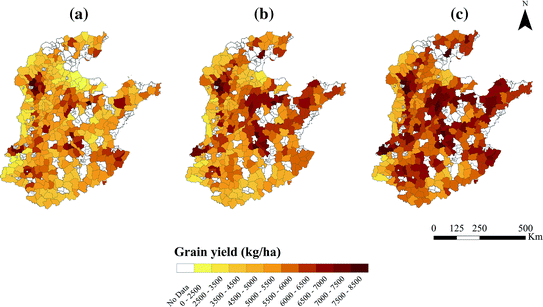
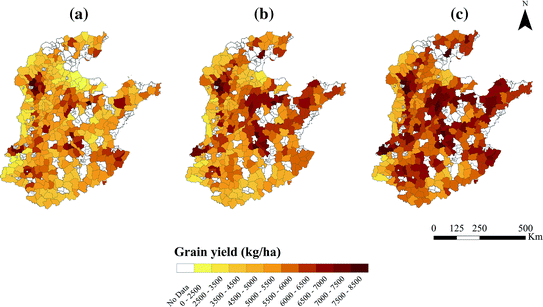
Fig. 2.2
County-level grain yield of the North China Plain, a 2000, b 2005, and c 2008
During the period of the year of 2000–2008, annual total grain production of the selected counties of the North China Plain increased by more than 40 %, and the average grain yield increased from 4994 to 5986 kg/ha (with an increasing rate of 20 %). The time-series changing trend of the total grain production is similar to that of the average grain yield, and therefore, the increase of agricultural production can be mainly ascribed to the increase in the average grain yield (Fig. 2.3). The time-series change of grain yield from the year of 2000 to 2008 can be divided into two periods. Firstly, the average grain yield decreased after the year of 2000 because of a decrease in grain price. Secondly, the price of grain increased again through governmental support after the year of 2003, which made the average yields recover again.
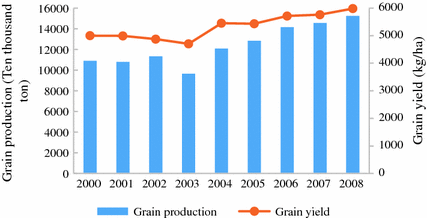
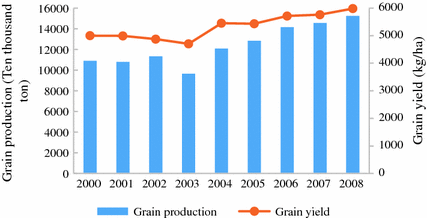
Fig. 2.3
Relationship between grain production and grain yield of the North China Plain, 2000–2008
Also, we showed that the application rates of fertilizer and pesticide increased with fluctuations from 525 to 622 kg/ha and from 12.6 to 16.2 kg/ha during the period of the year of 2000–2008, respectively. The application rate of the farm machinery also showed an increasing trend (Fig. 2.4). The increase of the application of farm machinery, fertilizer, and pesticide can be the key determinants of the increase in crop yield; however, further increases in fertilizer and pesticide application are unlikely to be as effective as they previously were in increasing the grain yields because of the gradually diminishing return rate.
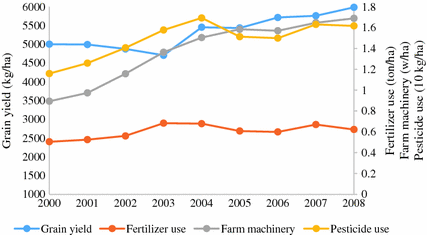
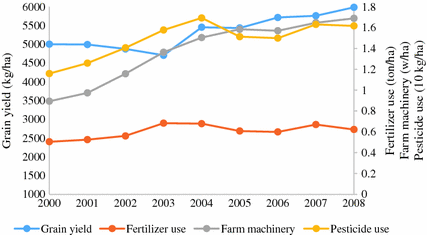
Fig. 2.4
Changes of grain yield, fertilizer use, pesticide use, and farm machinery application of the North China Plain, 2000–2008
Trends in NDVI. The annual average greenness (represented with NDVI) is chosen as the standard proxy of annual average biomass productivity, which generally fluctuates with the rainfall. The NDVI calculated using remote sensing image data, currently is one of the most commonly used indicators to monitor regional or global vegetation and the ecosystem, and is the best instruction factor to reflect vegetation growth conditions and coverage (Zhang et al. 2005). In this study, the cultivated land degradation areas were identified with a sequence of analyses of the NDVI data. The result shows that the annual average NDVI of the North China Plain showed an overall increasing trend during the year of 2001–2004. About 90 % of the study area’s annual average NDVI experienced an increasing trend. While only 5 % of the study area’s annual average NDVI showed a decreasing trend, which was distributed in the Shandong Province (Fig. 2.5a). In comparison, during the years 2004–2008, about 80 % of the study area’s annual average NDVI experienced a decreasing trend, with only 19 % of the study area’s annual average NDVI showed an increasing trend (Fig. 2.5b). Besides, the annual average NDVI in some areas showed first an increasing and then a decreasing trend during the year of 2000–2008. Overall, the annual average NDVI showed an increasing trend in most part of the whole study area during the year of 2000–2008 (Fig. 2.5c).
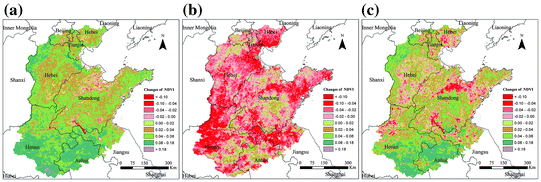
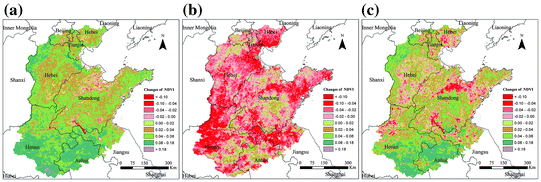
Fig. 2.5
Changes of NDVI of the North China Plain during a 2000–2004; b 2004–2008, and c 2000–2008
Land–use change. During the year of 2000–2008, the land-use change is mainly dominated by the conversion from cultivated land to other land-use types, especially the conversion from cultivated land to built-up land. As cultivated land is the major land-use types in the study area, thus we focus on the cultivated land conversion. In the North China Plain, cultivated land is mainly converted to built-up land (67 %), forest land (9.1 %), and grassland (12.4 %) (Fig. 2.6).
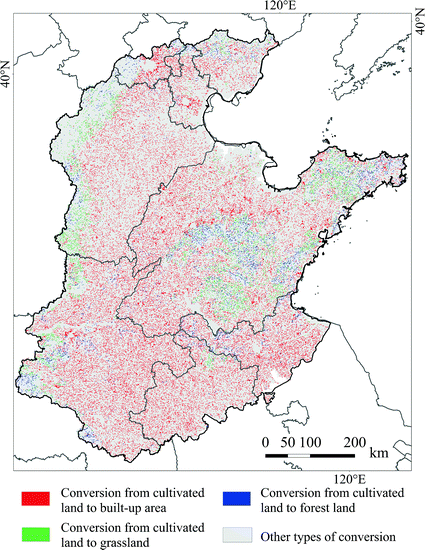
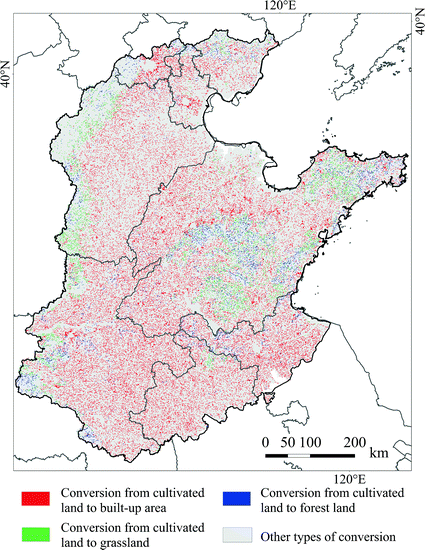
Fig. 2.6
Land conversion from cultivated land to three major land-use types during 2000–2008
Associations Between Climate Factors and Land Degradation
We investigated the relationship between NDVI (from the year of 2000 to 2008) and climate factors. The relationship between NDVI and climate indicators is widely analyzed in many previous studies, which have confirmed that vegetation changes are closely related to changes in the climate factors at the global or regional scale. In particular, it is widely acknowledged that the temperature and rainfall are the most significant factors influencing the ecosystem characteristics and distribution. We sampled the value of the NDVI, rainfall, and temperature and calculated their annual average values in the whole North China Plain. The mean NDVI, temperature, and rainfall for the study area were plotted along a nine-year time series (Fig. 2.7). Figure 2.7 visually shows that the average NDVI experienced a fluctuated increasing trend, and the average rainfall and temperature slightly showed an increasing trend with some fluctuations.
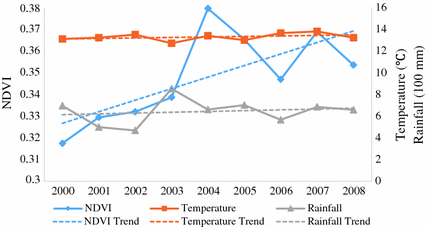
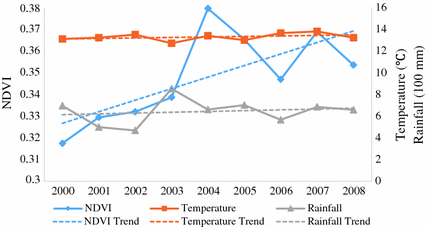
Fig. 2.7
Changing trend of spatially aggregated annual NDVI, rainfall, and temperature
Specifically, to study the responses of NDVI to the climate change, we analyzed the spatial correlation of NDVI with rainfall and temperature using the correlation analysis at the pixel scale. Figure 2.8 shows the spatial distribution of the correlation of NDVI with rainfall and temperature, respectively. It shows that the rainfall has a strong effect on NDVI, and there was a positive correlation between rainfall and NDVI in most part of the study area, especially in the northern part of the study area where it showed a high correlation. As to the relationship between NDVI and temperature, there was generally a positive relationship between NDVI and temperature, only in the northern part of the study area showed negative relationships.
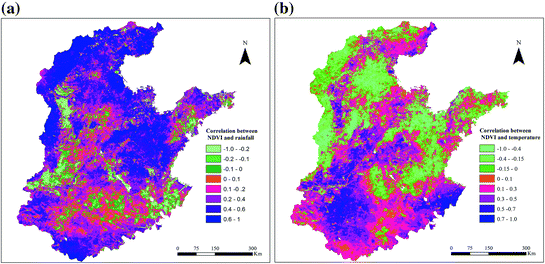
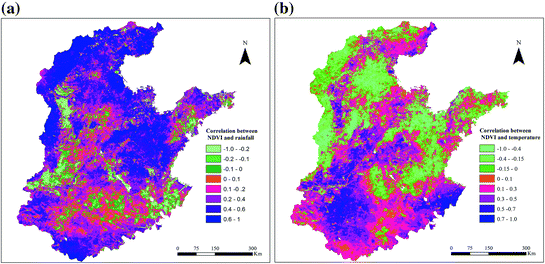
Fig. 2.8
Spatial patterns of correlation a between annual average NDVI and rainfall, and b between annual average NDVI and temperature at the pixel level in the North China Plain
Assessments of the Driving Forces of Land Degradation
The causes of land degradation are numerous, interrelated, and complex. The relationship between direct and underlying causes of land degradation is complex also, and the impact of underlying factors is context specific (Nkonya et al. 2011). Quite often, the same cause may lead to diverging consequences in different contexts because of its varying interactions with other proximate and underlying causes of land degradation. This implies that targeting only one underlying factor is not sufficient in itself to address land degradation. It is necessary to take into account a number of underlying and proximate factors when designing policies to prevent or mitigate land degradation. For our model specification, it is essential to identify the effects of various combinations and interactions of underlying and proximate causes of land degradation in a robust manner rather than only analyze the individual cause of land degradation. With this regard, we try to identify the key underlying and proximate causes of land degradation. In this part, we conducted econometric analysis of the causes of land degradation in the North China Plain with the binary logistic regression model.
We constructed the model for estimating causes of land degradation or land improvement at the county level, using annualized data, and the model specification is as follows:
Y t = 1, if NDVI t − NDVI t−1 >= 0 and Y t = 0 if NDVI t − NDVI t−1 < 0;

x 1t
a vector of biophysical causes of land degradation (e.g., climate conditions, topography, soil fertility constraints);
x 2t
a vector of demographic and socioeconomic causes of land degradation (e.g., population density, per capita GDP, rural farmers’ per capita income, and agricultural intensification);
x 3
a vector of variables representing access to infrastructure services (e.g., distance to expressway, distance to high way, and distance to other way);
x 4
a vector of variables representing land-use change (e.g., county-level percentage of land conversion from cultivated land to built-up land, forest land, and grassland during the year of 2000–2008)
Y t is a binary variable that being used to identify whether land degradation happens, if Y t equals or is larger than zero, then we assume that there happens land improvement; otherwise, there happens land degradation. Furthermore, we applied a binary panel logistic regression model to simultaneously study the relationship between land degradation conditions and all other variables. Table 2.1 illustrates the results of the regression. The results are to be interpreted with extreme caution due to the complex and multidirectional relationship between NDVI changes and the selected variables. Note that the results might not indicate a causal relationship but only an association between NDVI and the selected biophysical and socioeconomic variables (Nkonya et al. 2011).
Table 2.1
Results for binary panel logistic regression analyses for the biophysical and socioeconomic driving forces of land degradation
Land degradation conditions Y (1 represents land improvement and 0 represents land degradation) | Coef. |
---|---|
△Rainfall (mm) | 3.221*** |
△Temperature (°C) | 1.025*** |
Percentage of land conversion from cultivated land to built-up land (%) | −0.326 |
Percentage of land conversion from cultivated land to forest land (%) | 1.788 |
Percentage of land conversion from cultivated land to grassland (%) | 8.507* |
△Fertilizer utilization per unit area (kg/ha) | 0.506** |
△Population density (ten thousand people/km2) | −11.282 |
△Rural farmers’ per capita income (ten thousand yuan) | −0.076 |
△Per capita gross domestic production (ten thousand yuan) | 0.0004 |
△Share of production value of agriculture, animal husbandry, and fishery in GDP (%) | −1.672* |
DEM (km) | 3.214 |
Slope (degree) | −0.018 |
Soil organic matter (%) | 0.446*** |
Distance to highway (km) | 0.002* |
_cons | 0.032 |
Climate change is significantly related with the land degradation conditions which represented by the changes in NDVI. As expected, increments in temperature and rainfall are positively related with the increases in NDVI, and increases in temperature and rainfall will significantly contribute to land improvement. Land-use change is also the major driving force of land degradation. The results showed that the conversion from cultivated land to grassland and forest land will contribute to land improvement, which corresponded to increase in NDVI, while the conversion from cultivated land to built-up land would lead to land degradation. Among the land conversions, only the conversion from cultivated land to grassland significantly affects the land degradation. In comparison, climate change plays a much more important role than land-use change in land degradation. Besides, agricultural intensification represented by fertilizer utilization is significantly and positively related with land improvement, as fertilizer application increases soil carbon (Vlek et al. 2004), which could correspond to an increase in NDVI. Increases in the population density would lead to land degradation, but not significantly. According to the previous studies, the impact of population density on land degradation is ambiguous, while our results suggested that there would be more serious land degradation in areas with higher population density. The population growth will lead to increasing demand for housing and other facilities, which in turn can lead to increase of impervious surface as a result of urban development and infrastructure construction and deforestation. Rapid economic development, which can be represented by changes in the per capita GDP, shows that an increase in both GDP and NDVI had positive impacts on land quality, suggesting the role of ecosystem service could play in economic growth and prosperity. Economic development would stimulate the land improvement, but such impacts were not significant, while the rural economic development, represented by rural farmers’ per capita income, showed negative relationship with land improvement, suggesting that the NDVI will decrease as the rural farmer’s income increases. It may be due to the fact that rural economic growth develops at the cost of intensive land-use practice, which contributes to environmental degradation further lead to the land degradation. In addition, the increases in the share of the primary industry (agriculture, animal husbandry, and fishery) in GDP significantly corresponded to the decrease in NDVI, as production value of the agriculture increases were mostly resulted from the investment into agricultural production, such as fertilizer, pesticide, and farm machinery, not from natural land-quality improvement. In addition, the geographic and topographic factors also affect the land degradation. As to the topographic factors, the results showed that the higher the elevation and the slower slope, the less serious land degradation. The impacts of elevation was not significant, while it may suggest that in the areas with higher elevation, there would be less impact of anthropogenic factors on the land degradation. The slope had clear significant impacts on land degradation, suggesting steep slopes would lead to land degradation more easily, as steep slope region was more vulnerable to severe water-induced soil erosion. As to the soil fertility represented by SOM, the results showed that the higher the soil fertility was, the less possibility of land degradation. Besides, the distance to highway was significantly positively related with land improvement, which suggested that the larger distances to road network meant there was less human disturbance, less infrastructure development and land-use change, which would exert less impact on the land degradation.
Summary
Land degradation or improvement is an outcome of many highly interlinked direct and underlying causes, including natural, socioeconomic, and related agricultural practices. In China, many types of land degradation are occurring, such as grassland degradation, deforestation, and cultivated land degradation. In this study, we conducted an empirical study in the North China Plain and analyzed the basic grain production changes, the changing trend of NDVI, and the spatial pattern of relationship between climatic factors and NDVI. Further taking NDVI as a proxy for land degradation and improvement, we applied an econometric model to analyze the causes of land degradation.
According to the analyses, both the grain production and grain yield of the North China Plain showed an increasing trend during the year of 2000–2008; however, estimation based on the remotely sensed NDVI data showed that the North China Plain still experienced land degradation. Degraded areas have been expending in the northern part of the study area, though many parts of the study area showed land improvement. Based on the correlation analysis, the increases in temperature and rainfall corresponded to the increase in NDVI in most parts of the North China Plain, though some parts of the study area showed negative correlation between NDVI and temperature and rainfall. Further, according to the binary panel logistic regression analysis, the results showed there were strong impacts of some key biophysical and socioeconomic variables on land degradation. Some of these relationships were consistent with conclusions in previous case studies, while other showed complex differences. The results for climate factors were not surprising; increase in temperature and rainfall would contribute to land improvement. Different land-use changes exert different impacts on land degradation. Unsurprisingly, increasing of forest land and grassland would benefit land quality, while urban expansion would lead to land degradation. Our estimation results about the positive effects of agricultural intensification represented by fertilizer utilization on land degradation were also as expected, and the SOM was also a crucial positive factor for land-quality conservation. Rural economic and agricultural development may lead to land degradation. The rural economic and agricultural production growth exerted negative impacts on land quality, which means the development of rural economy and agricultural production led to land degradation. The increases in rural farmers’ per capita income and primary production value ratio did not result in the improvement of land quality, which may be due to the overexploitation of land with insufficient investment into the land conservation. With this regard, an effective response to land degradation needs increasing incentives of farmers to conserve their cultivated land and improve their access to the knowledge and inputs that are required for proper conservation. Promotion of such land improvements should be a development policy priority. During the process to promote rural economic development, the governments should also focus on the monitoring and assessment of land quality and make measures to improve land quality, and such improvement measures should be designed together with farmers to meet their prior needs and use appropriate techniques according to the local economic and social conditions. In addition, increasing accessibility to infrastructures may also have negative effects on land quality. Infrastructure development is the basis for regional prosperity, and a booming economy will result in more construction of infrastructure. The expansion of basic infrastructure of transportation such as roads, railways, and airports can further take up land resources and further result in land overexploitation and degradation. The covering of the soil surface with impervious materials as a result of urban development and infrastructure construction is known as soil sealing. Sealing of the soil and land consumption are closely interrelated, when natural, seminatural and cultivated land is covered by impervious surfaces and structures; this will degrade soil functions or cause their loss. To reduce the impacts of infrastructure construction on land quality, the local government should take the assessment of land degradation into consideration during the construction of infrastructures.
Based on the results of the above analysis, to achieve sustainable land management, climate change should be monitored so as to make adaptation measures to mitigate the impacts of climate change on land quality; along with the socioeconomic development, investments and better land management for improving land quality should be definitely encouraged through appropriate policy measures; human activities that change the land surface, such as infrastructure constructions, should be regulated on the basis of the assessment of impacts on land quality, and corresponding land conservation measures should be taken during the construction process.
Predicted Land-Use Conversions in the North China Plain
Introduction
Land-use change, as the direct cause and response of regional environment change, has always been one of the core topics of global change research (Foley et al. 2005). It is difficult to analyze the relationship between land-use and climate change clearly. On the one hand, climate change should exert impacts on the production of cultivated land, forestry, grassland, and so forth. For example, agricultural yields can be directly affected by climate change through changing temperature and precipitation, the distribution of pests, and the frequency of forest fires, and the markets can also be affected by climate change (Haim et al. 2011). Moreover, in recent years, there have been a number of literature-analyzed effects of climate change on agricultural production, with the help of some models (Schlenker et al. 2005; Jiang et al. 2012b). From these pieces of literature, we can learn that hedonic price models are widely used to estimate the relationship between county-level farmland values and climate variables such as temperature and precipitation. These models are then used to simulate the effects of climate change on the value of agricultural production (Deschenes and Greenstone 2007). Even so, some researchers think that it is unreasonable to use mean temperature in the analysis of climate change impacts on agriculture (Schlenker and Roberts 2009). They find that the grain output has a good positive correlation with temperature but then falls quickly as temperature increases above a certain threshold. Other studies support these findings for global timber productivity (Sohngen et al. 2001; Perez-Garcia et al. 2002). In most regions, crops are sensitive to climate change (Izaurralde et al. 2003; Lobell and Field 2008). Usually, global warming will accelerate the crop development, alter the growing season, and enhance the maintenance respiration (Qu et al. 2013), while, as to animal husbandry development, global warming would lead to grassland degradation, which would reduce the amount of livestock grazing and then decrease the output of livestock production. Meanwhile, livestock grazing might promote the CO2 emissions (Herrero et al. 2009). According to the researches mentioned above, climate change will affect the agriculture, forestry, animal husbandry production, and so forth; then, the demand for products of different land-use types can result in new balance of the supply of and demand for land and further lead to land-use structure changes. On the other hand, most of experts think that the climate system should take account of land use; that is, land-use/land-cover change plays an important role in climate change; for example, afforestation and reforestation can reduce atmospheric CO2 concentrations, thus, to mitigate the emission of greenhouse gas, and deforestation can result in temperature rising and precipitation decreasing in some regions (Nobre et al. 1991). Then, through the connection of climate change, we can figure out that the land-use structure has relationships with climate change.
Research on land-use structure changes needs to identify the driving factors from a systemic perspective and choose an appropriate model as a tool to reflect the changes to spatial distribution on a certain scale (Deng et al. 2008b). At present, there have been some achievements in applying economic models and empirical statistical methods for analyzing the driving forces of the land-use structure changes, and the simulation about land structure change has a tendency of regionalization and of being microcosmic, while there is much more room for improvement in many links of such simulation researches (Deng et al. 2012). For example, quite a lot of case studies just simulated the changes of one or several types of land use and seldom comprehensively simulated macroscopic structural changes of all land-use types from a systemic perspective (Capozza and Helsley 1989). The change of land-use structure is a dynamic process, which should be based on regional socioeconomic development characteristics, cultural traditions, natural conditions, the previous trend of land-use structure changes, and other factors to acquire valuable simulation results for decision. And it is helpful to make the forecast and evaluation results more science oriented and rational by simulating regional land-use structure changes under different scenarios.
The North China Plain is located in 32–40°30′N, 113–120°30′E, which belongs to the warm temperate zone, with the flat terrain, deep soil layer, and peaks and valleys of rainfall and heat in the same period. It covers seven provinces and cities, including Hebei, Henan, Shandong, Jiangsu, Anhui, Beijing, and Tianjin (containing 387 counties), and has an area of 33.4 million ha, among which the area of land is 21 million ha, and it is the largest plain in China. The region with convenient transportation developed industries, and sufficient labor is one of the most important agricultural regions, and its agricultural production has a great potential for development. The North China Plain is a region that was earlier developed and was greatly influenced by human activities, and it is also one of the economically developed regions in China. The distribution of towns and cities is relatively intensive in the North China Plain; in addition to Beijing and Tianjin, there are also more than 20 cities with a population of more than one million. In the North China Plain, the grain output accounts for 18.4 % of total national output; the output of cotton accounts for 40 % of total national output; and the output of oil-bearing crops also accounts for a large proportion in China.
In 2006, the GDP per capita (GDPPC) in the North China Plain reached 19,224.27 Yuan. According to the experience of industrialization and urbanization in the developed countries and districts, the urbanization development of the North China Plain has entered into the period of cluster development and the later period of industrialization development. Since the North China Plain is located in a vast plain area and the land-use types are relatively unitary, the core problem of land use lies in the contradiction between the cultivated land protection and nonagricultural construction land (Deng et al. 2008b). The North China Plain is one of the important commodity grain bases in China, while its built-up area’s expansion has taken up a large amount of high-quality cultivated land resources, which poses threat to regional and national food security and ecological environment.
In the North China Plain, most researches focused on the impact of climate change on cultivated land. There are few researches of the response mechanism on grassland and built-up area, and only a few studies have explored the combined effects of climate change on the agricultural and forestry sectors. The North China Plain is China’s traditional and important agricultural production base, and it is also a region where the population grows rapidly; economy and urbanization develop rapidly with obvious land-use conversion, especially the transfer between cultivated land and nonagricultural land use (Jiang et al. 2012a). Therefore, in this study, we selected the North China Plain as case study area to analyze the future land-use structure changes under different scenarios. And based on an econometric model, we analyzed the effect of natural conditions (climate factors such as precipitation, temperature, etc.) and socioeconomic factors on land-use structure changes. This study can provide reference for the decision making of the local land-use planning, urbanization management, and land-use management.
Land-Use Change in the North China Plain
In this study, the information about land-use structure and its changes was derived from the remote sensing images of the North China Plain in years 1988, 1955, 2000, 2005, and 2010 though the man–computer interactive interpretation (Table 2.2). And the information can be used as the basic data for analyzing the driving mechanism of land-use structure changes.
Table 2.2
Land-use structure of the North China Plain in various years (unit ten thousand hectares)
Land-use type | 1988 | 1995 | 2000 | 2005 | 2010 | Change rate during 1988–2010 (%) |
---|---|---|---|---|---|---|
Cultivated land | 2448.81 | 2410.86 | 2402.01 | 2372.28 | 2346.51 | −4.18 |
Forestry | 467.01 | 472.01 | 470.82 | 471.05 | 471.48 | 0.96 |
Grassland | 375.12 | 363.34 | 363.48 | 356.25 | 355.51 | −5.23 |
Water area | 310.09 | 312.74 | 315.28 | 317.84 | 321.02 | 3.53 |
Built-up area | 1137.88 | 1187.64 | 1197.93 | 1237.09 | 1263.32 | 11.02 |
Unused land | 40.20 | 32.50 | 29.58 | 24.59 | 21.25 | −47.12 |
According to the statistics in Table 2.2, in the North China Plain, cultivated land accounts for the largest share about 50 %, and built-up area, forestry, water area, and grassland overall occupy a certain proportion, which guaranteed the development of local farming, forestry, animal husbandry, side-line production, and fishery. From 1988 to 2010, there had been some changes in the macrostructure of land use; cultivated land and grassland decreased by 4.18 and 5.23 %, respectively. And along with the development of economy and expansion of urbanization, the built-up area increased by 11.02 % from 1988 to 2010. In addition, the change trends of each land-use type during 1988–2010 are also not the same; cultivated and unused land always showed a trend of decrease, while water area and built-up area showed a continual trend of increase. The forestry overall increased with interval decrease from 1995 to 2000, and the grassland overall decreased with a slight interval increase from 1995 to 2000.
Data and Methodology
Data
The data used in this study include the basic geographic information data, socioeconomic data, and climate data that can affect land-use structure changes. The basic geographic information data mainly include remote sensing data and biological geographic elements data. And the land-use data and climate information are uniformly rasterized into 1 km × 1 km grid cell. The land-use data mainly come from remote sensing images interpretation during 1988–2010 (Yin et al. 2010), and then, the information of interpreted raster data can be extracted from county level. And thus, we derived the geographic elements information of each county, including temperature and precipitation. The concrete operations of data are mainly based on ArcGIS 9.3 and STATA 10.0 platform, and the data eventually were prepared in the form of panel data for analyses (Deng et al. 2012).
In this study, the socioeconomic data and climate data of 378 counties in the North China Plain were collected in 1988, 1995, 2000, 2005, and 2010 (Table 2.3). However, the land-use data are of spatial data type. To make the land-use data match with the statistic data of socioeconomic factors, the land-use data should be aggregated into county level. And in order to increase the sample space, improve the degree of freedom, provide individual information, and make the estimated results more accurate, the data mentioned above were prepared into the format of panel data which integrated the cross-sectional data and the time-series data.
Table 2.3
Descriptive statistics of main variables at county level